Have you ever wondered what happens to your personal data once it enters a company's system ? From your passport details to that cute puppy picture you uploaded last week, how do organizations ensure different types of information receive appropriate protection ?
The Privacy Puzzle: More Than Just Keeping Secrets
In today's digital age, privacy has evolved beyond simply keeping information confidential. It's about giving users control over their data - how it's used, where it's stored, and who has access to it. But before companies can implement effective privacy controls, they need a fundamental understanding of what data they possess and where it resides.
The Foundation: What is Data Classification?
Data classification serves as the cornerstone of any robust privacy framework. Think of it as creating a detailed map of your organization's data landscape. Just as a librarian categorizes books by genre and subject matter, companies must organize their data by type and sensitivity level. To build privacy controls, companies need to know what and where user data is. Companies can only build technical controls and processes to delete or change user data if they can find it. That's where data classification comes in. This systematic approach ensures that each piece of information receives appropriate handling and protection.
Building Your Data Classification Framework
The Tools of the Trade
In the modern digital ecosystem, manual classification is no longer sufficient. Companies need useful tools to manage their vast data repositories effectively. The most important is to start early on.
Here's what's typically in a privacy professional's toolkit, but there are more ways to do it :
- Automated Tagging Systems
- Automatically identify and label data types
- Reduce human error in classification
- Enable scalable data management
- Data Schemas
- Provide structured frameworks for data organization
- Ensure consistency across different systems
- Facilitate easier data tracking and management
- Data Scanning Tools
- Continuously monitor data flows
- Identify potential privacy risks
- Flag misclassified information
While companies can use manual tools to classify data, it is preferable to automate where possible. Human interaction increases the likelihood of human error. It is much, much harder to build data classification processes and tools after there are established processes and a large data footprint.
The Risk Matrix: Understanding Data Sensitivity
The Tools of the Trade
Not all data is created equal. A fundamental principle of effective data classification is understanding the varying levels of risk associated with different types of information.
For example, users could upload their personal information like a ID. They could also upload something less sensitive such as a picture of their garden. The best way is to visualize it this way, if there is a data breach, there are more negative consequences for ID information being compromised than a garden picture. That´s why data classification and risk go hand and hand. This is the reason why data classifications should have predetermined risk levels assigned to them. Therefore, the risk levels determines the type of security and privacy controls applied.
Let's break down the standard risk levels:
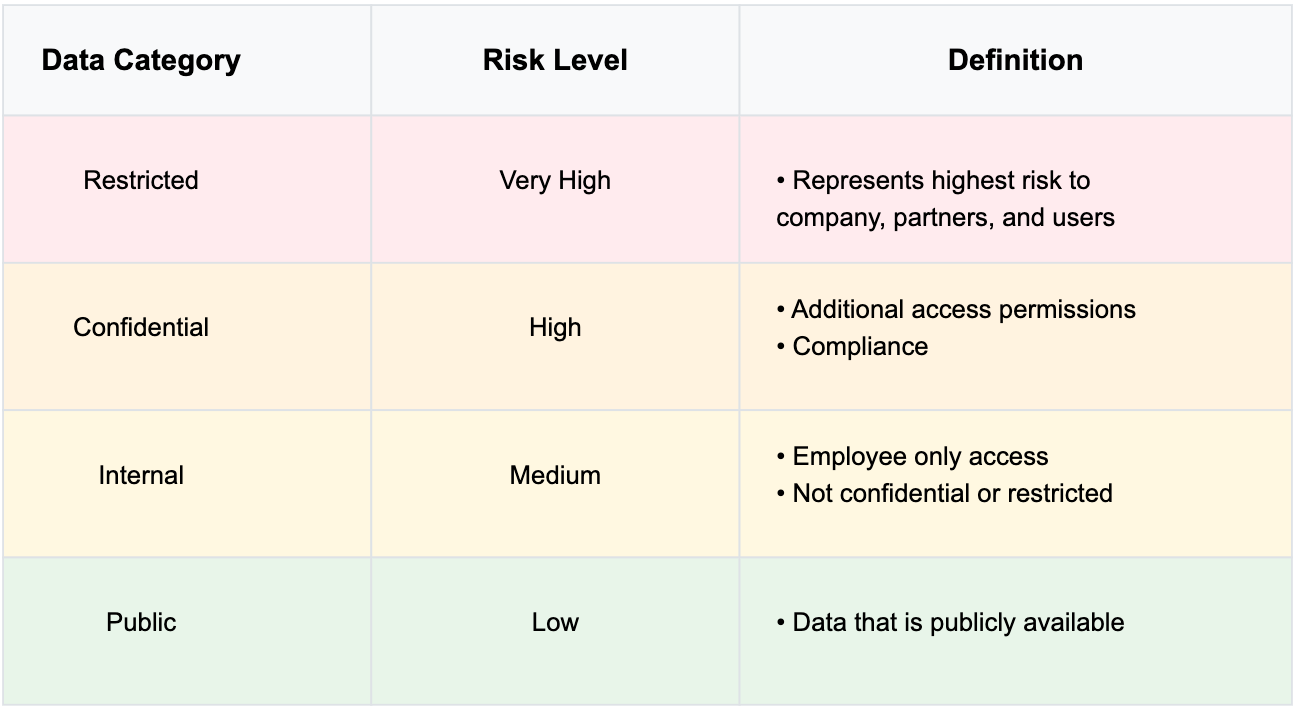
Understanding Data Risk Classifications: A Strategic Framework
Let's explain this above typical four-tier classification model that most enterprises adopt:
Public Data (Low Risk)
Think of this as your company's "open book" – information that's already in the public domain or intended for public consumption. This might include press releases, public financial reports, or marketing materials. While still requiring proper management, these datasets pose minimal risk if shared.
Internal Data (Medium Risk)
This category encompasses day-to-day operational information shared within the organization's walls. While not highly sensitive, this information is meant for employee eyes only, accessible to staff members and trusted partners under NDAs. Examples might include internal procedures, team communications, or non-sensitive project documentation.
Confidential Data (High Risk)
Moving up the sensitivity ladder, confidential data requires special handling and stricter access controls. This tier often involves information subject to regulatory compliance or business-critical data. Access is granted on a need-to-know basis, with additional verification steps and permissions required. Think client records, financial data, or strategic business plans.
Restricted Data (Very High Risk)
At the summit of the risk pyramid sits restricted data – the crown jewels of organizational information. A breach at this level could spell disaster, potentially resulting in severe financial repercussions, legal consequences, lasting reputational damage, loss of competitive advantage, compromise of user privacy.
Examples might include trade secrets, sensitive personal information, or critical infrastructure details.
This data risk framework serves as a foundation – organizations often customize these classifications based on their unique risk landscape, industry requirements, and regulatory obligations. The key lies in implementing consistent, clear criteria for each level while maintaining flexibility to adapt to evolving threats and business needs.
Implementing Your Classification Strategy
Best Practices for Success
- Start Early The earlier you implement data classification processes, the better. Retrofitting classification systems onto existing data structures can be complicated and resource-intensive.
- Automate Where Possible While human oversight is important, automated systems reduce errors and increase efficiency. They provide consistent classification across large datasets.
- Regular Review and Updates Privacy requirements and data usage patterns evolve. Regular reviews ensure your classification system remains effective and compliant.
The Impact of Proper Data Classification
Consider this scenario: A company experiences a data breach. The difference between a minor incident and a major crisis often lies in how well the affected data was classified and protected. While a leaked public dataset might cause minimal disruption, compromised restricted data could lead to:
-
- Significant financial penalties
- Legal consequences
- Lasting reputational damage
- Loss of customer trust
- Compromise of user privacy
Taking Action: Next Steps for Your Organization
Ready to strengthen your data privacy framework?
Here are your next steps:
- Audit your current data inventory
- Identify gaps in your classification system
- Implement appropriate tools and processes
- Train your team on new procedures
- Regularly review and update your framework
Remember:
Privacy protection starts with understanding what you're protecting. By implementing robust data classification, you're not just checking a compliance box - you're building a foundation for sustainable data privacy management.
Learn more about Privacy and Data Protection with us on this blog.
Source: "AI Governance in Practice Report 2024" IAPP