Today´s discussion
Third-Party AI Assurance: Building Trust in the Age of Artificial Intelligence
VALIDATING AI SYSTEMS THROUGH INDEPENDENT EXPERTISE
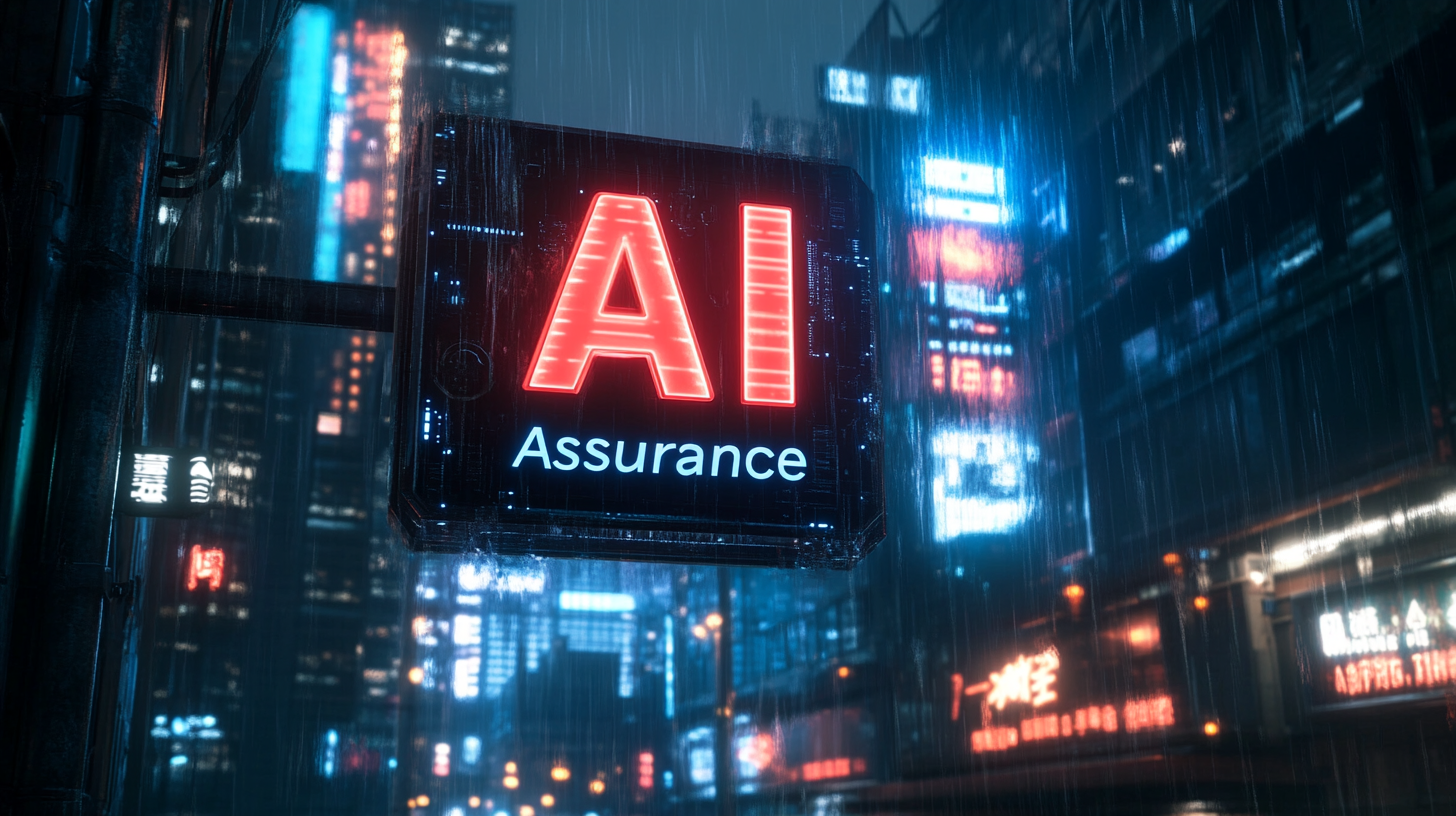
Organizations worldwide are grappling with a critical question: how can they demonstrate their AI systems are trustworthy and accountable ? The answer lies in robust AI assurance methods.
What is AI Assurance ?
In this UK report of "Guidance Introduction to AI assurance", assurance is defined as "the process of measuring, evaluating and communicating something about a system or process, documentation, a product, or an organisation."
Why is AI Assurance so Important ?
Third-Party AI Assurance
What is Third-Party AI Assurance ?
> Assessments
> Testing and Validation
> Conformity Assessments
> Impact Assessments
> AI/Algorithmic Auditing
> Certifications
Today, organizations face a critical challenge : ensuring their AI systems are trustworthy, ethical and compliant. We´ve seen how third-party AI assurance provides essential validation mechanisms for responsible AI deployment.
As AI transforms industries with potential economic benefits, the need for robust assurance frameworks has become paramount. Third-party validation complements internal expertise through various mechanisms: detailed assessments identify risks and biases, specialized testing validates performance and fairness, conformity checks ensure regulatory compliance, and impact assessments evaluate implementation contexts.
The emergence of standardized AI auditing frameworks, though still evolving, signals a shift toward more structured evaluation methods. This trend, coupled with growing regulatory emphasis on independent verification, makes third-party assurance not just beneficial but essential. Certifications serve as trust markers, helping organizations demonstrate their commitment to responsible AI while providing stakeholders with clear evidence of compliance.
Want to read more about AI Governance, look at our blog articles and contact us to receive our advises to implement a Responsible AI framework in your company.
Source : IAPP AI governance in practice 2024 report.