Today´s discussion
Investing in AI Security Drives 35% Revenue Growth
Gartner's Roadmap to Success

Is your organization prepared for the evolving security landscape of enterprise AI systems ? With nearly 30% of enterprises experiencing AI security breaches, the need for robust governance and security frameworks has never been more essential for companies.
The Growing Challenge of AI Security
In its last webinar, Gartner research´s Avivah Litan and Jeremy D´Hoinne reveal an upcoming trend for AI systems : AI security is now the board's top priority, driving unprecedented focus on governance and security funding. This shift comes as companies are facing new types of threats: from data compromise by internal parties (62%) to external attacks (51%) on AI infrastructure.
Why Traditional Security Approaches Fall Short ?
AI systems present unique security challenges that transcend traditional cybersecurity measures. Modern AI applications are more than just models : they encompass complex ecosystems of data retrieval, prompt engineering and runtime environments. This complexity creates new attack surfaces that traditional security tools aren't designed to address.
Potential AI risks and liabilities can arise from the use of AI systems, especially in areas like facial recognition, conversational AI, and deepfakes. It is essential to have robust governance, security and risk management practices in place to mitigate these types of AI-related incidents to face this real-life examples:
-
Wrongful imprisonment: facial recognition technology led to the wrongful imprisonment of a person in Louisiana for a week.
-
Chatbot liability: an Air Canada chatbot provided a passenger with an incorrect discount for a bereavement flight and the airline was held liable for the chatbot's actions.
-
Fraud scam: in Hong Kong, hackers used deepfake technology to impersonate a finance officer and trick a company into transferring $25 million to a fraudulent account.
Why Attack Surfaces Expand Across GenAI Life Cycle ?
The shift to Gen AI introduces new attack vectors that organizations must address through a comprehensive security and risk management approach across the entire lifecycle of these AI systems:
-
Prompts and prompt injection: With GenAI, the prompts used to generate the model outputs become a new attack vector. Malicious actors can attempt to inject harmful prompts to manipulate the model's responses.
-
Runtime risks: When running GenAI models, either your own or third-party models, there are new runtime risks to consider. Attackers may try to compromise the runtime environment to gain access or control over the model.
-
Data orchestration: The data used to train and fine-tune GenAI models becomes a critical attack surface. Adversaries may try to poison or compromise this data to impact the model's behavior.
-
Third-party models and libraries: Many organizations leverage pre-trained models or open-source libraries when building their own GenAI applications. These third-party components introduce new supply chain risks that must be accounted for.
-
Expanding attack surface: Unlike traditional software, the attack surface for GenAI expands beyond just the application code. It now includes the prompts, runtime environment, training data, and third-party dependencies - all of which require careful security consideration.
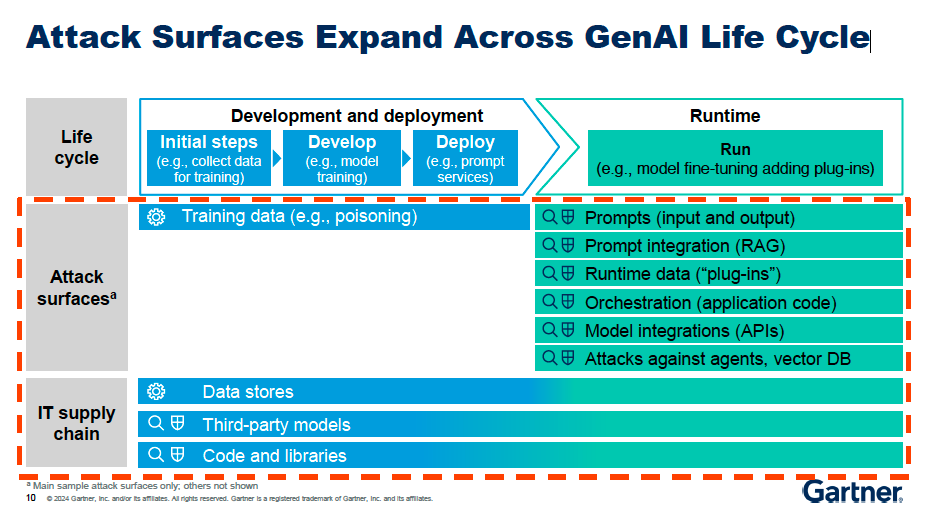
Key Issue Take-Away:
"GenAI has highlighted the need at the board level for AI governance and security funding. Most companies still have a lot of work to do when it comes to policies and governance."
The 3 AI Systems Initiatives
There are 3 main types of AI initiatives that organizations need to consider:
-
Out-of-the-box AI applications:
- These are top 50 AI applications like ChatGPT, Microsoft Copilot, Salesforce Einstein, etc.
- The key focus here is on protecting employees and the organization from the risks of using these applications, such as:
- Shadow IT (unauthorized use of AI apps)
- Ensuring acceptable use policies are defined and enforced
-
Embedded AI applications:
- These are AI capabilities integrated into existing enterprise applications like Microsoft 365, Salesforce, ServiceNow, etc.
- The approach is to identify the new AI-powered features and audit access controls before enabling them.
- Careful monitoring of the data processed and outputs generated is required.
-
Building own AI systems:
- For organizations building their own AI applications and models, the security and risk management approach differs:
- You have more control over the application stack and model, but need to secure the entire pipeline.
- Securing the retrieval, augmented generation, and prompt engineering workflows is crucial.
- Hardening the model against adversarial attacks and managing model drift are important.
- For organizations building their own AI applications and models, the security and risk management approach differs:
Regardless of the approach, a comprehensive framework like Gartner MOST (Mitigate, Observe, Secure, Trust) is recommended to address the evolving compromise vectors and risk management measures specific to AI.
The MOST Framework: A New Approach to AI Security from Gartner
Gartner's Model Operations, Security and Trustworthiness (MOST) Framework provides a comprehensive approach to managing AI risks:
- Model Management: Ensuring AI model integrity and performance
- Operations: Monitoring and maintaining AI systems effectively
- Security: Protecting against new types of attacks specific to AI
- Trustworthiness: Addressing ethics, bias mitigation and enterprise risk management
How to Determine How to Manage Risk ?
The way you deploy Generative AI will determine how you manage the associated risks
The key is to understand where your company fall on the spectrum and invest in the appropriate security, risk and privacy tools to address the unique challenges introduced by Generative AI in your environment:
-
Consuming everything from a provider: if you are using an "out-of-the-box" Generative AI application from a vendor (e.g. Microsoft Copilot, Salesforce Einstein, etc.) there is limited control you have over managing the risks.
The provider may not offer tools to:- Detect content anomalies in inputs and outputs
- Protect sensitive data from being compromised or leaked
- Verify the accuracy and trustworthiness of the Generative AI outputs
-
Building your own generative AI applications: if you are building and deploying your own Generative AI models and applications, you have more control and responsibility over managing the risks.
This includes:- Ensuring model transparency and explainability
- Hardening the models against adversarial attacks
- Implementing model performance monitoring and drift management
-
Hybrid approach: most organizations today fall somewhere in the middle: consuming some Generative AI from vendors while also building their own applications. This requires a combination of managing risks from both perspectives.
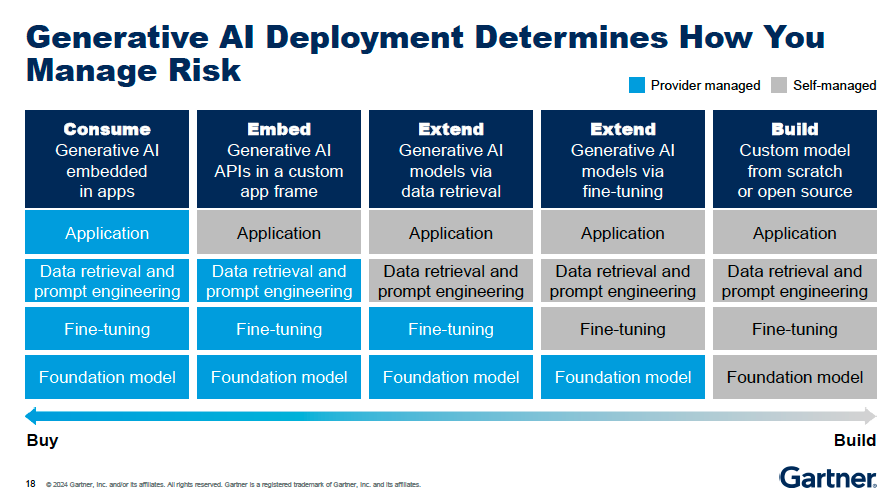
Apart from the Challenges to Securing AI Systems
Companies face several challenges in securing their AI systems:
- Data classification delays: most companies struggle with proper data classification
- Shadow AI risks: unauthorized AI applications pose significant security threats
- Inadequate content filtering: traditional DLP solutions don't address context-based filtering needs
- Governance uncertainty: many organizations have committees but lack clear policies
Apart from these situations, they need to take into account :
The 3 Main Gaps in Hosting GenAI Provider Controls
While hosting providers offer the core GenAI infrastructure, organizations need to supplement these services with additional security, risk and trust management tools and capabilities to fully address the unique security challenges of GenAI:
-
Content anomaly detection for Input/Output risks: hosting providers do not currently offer tools to detect anomalies in the inputs and outputs of GenAI models. This is needed to enforce acceptable use policies and identify potentially harmful or biased responses.
-
Data protection and obfuscation: hosting providers do not provide capabilities to obfuscate sensitive or confidential data when it is accessed by GenAI models. This is required to protect PII and other sensitive information.
-
Application security: hosting providers do not monitor the client-side security of GenAI applications, such as protecting against prompt injection attacks, API attacks or unauthorized access to model parameters. Organizations need to implement these controls themselves.
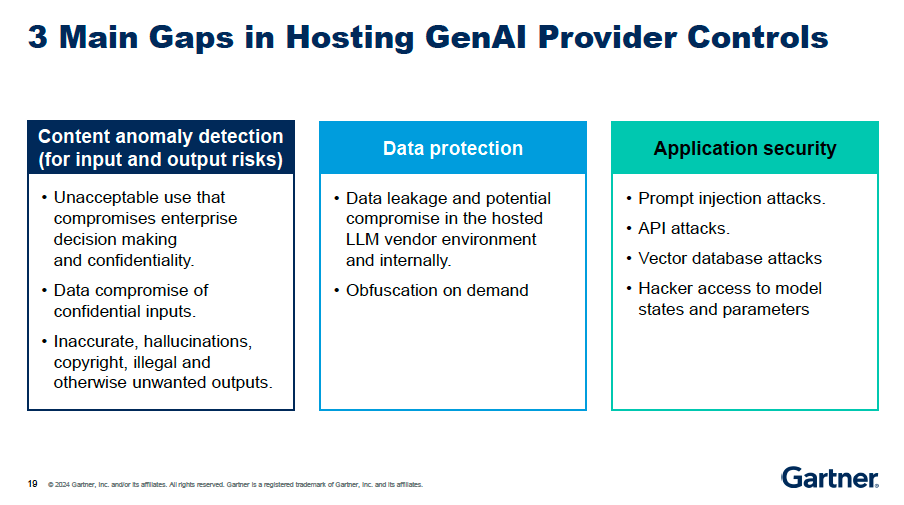
The Gartner TRiSM Solution
To face those gaps, Gartner developed TRiSM framework (Trust, Risk, and Security Management) for AI that provides a comprehensive approach to managing the challenges associated with deploying AI systems. So companies can build a more robust and trustworthy AI infrastructure, mitigating the risks while unlocking the full potential of these transformative technologies.
What is the TRiSM framework :
-
Trust: ensuring AI models are transparent, explainable and accountable. This includes managing the model lifecycle, monitoring for performance drift and mitigating biases.
-
Risk: identifying and addressing potential risks such as data privacy violations, security breaches and hallucinated or inaccurate outputs. This requires robust anomaly detection and content validation.
-
Security: hardening the AI application stack against attacks like prompt injection, API exploitation, and model parameter tampering. Implementing secure development and deployment practices is relevant.
The key aspects of the TRISM framework include:
- Adopting a hybrid approach with centralized governance and distributed ownership across teams (ex: security, data, AI, compliance).
- Implementing a comprehensive set of tools and controls to address the unique security, risk and trust challenges of AI systems.
- Establishing clear policies, processes and accountability measures to manage AI risks throughout the lifecycle.
- Fostering cross-functional collaboration and knowledge sharing to effectively navigate the complexities of AI deployment.
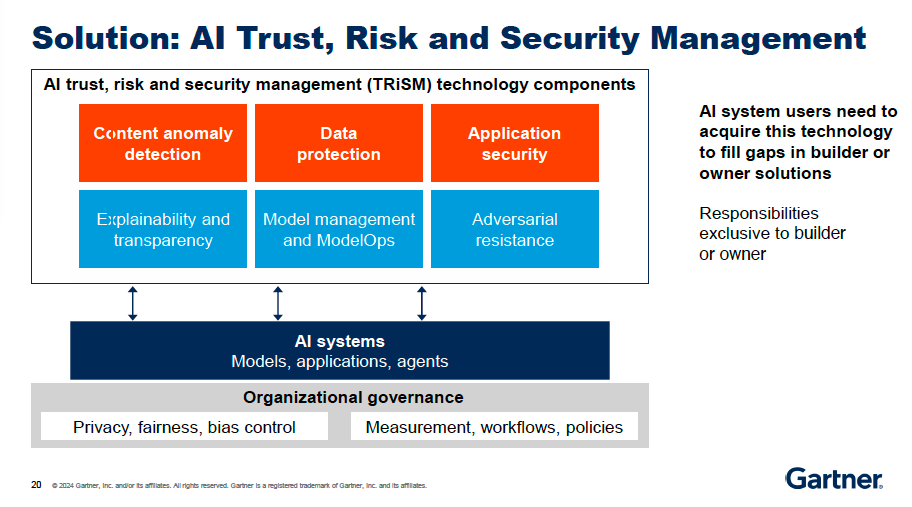
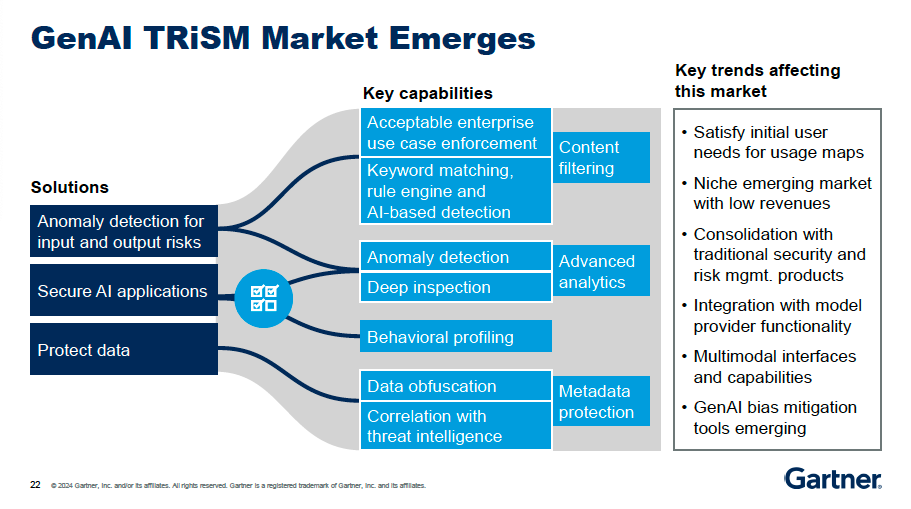
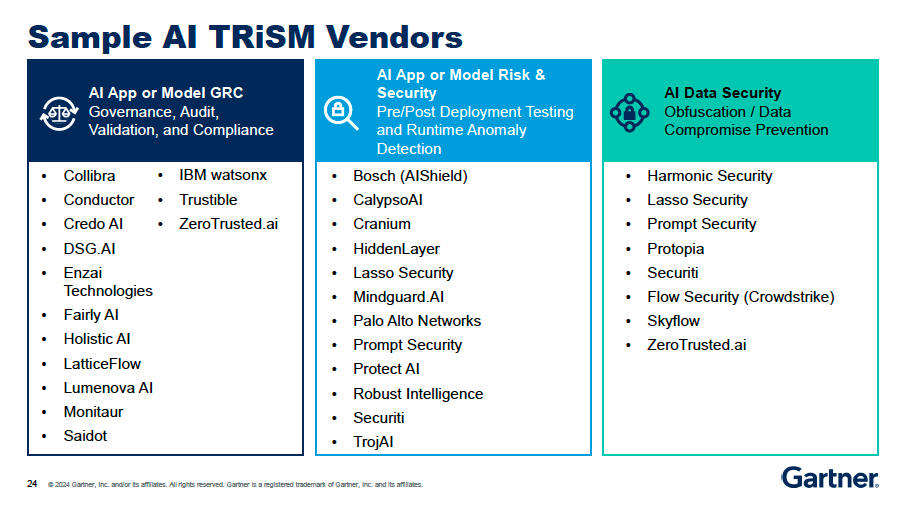
"By 2026, GenAI will cause a spike in the cybersecurity resources required to secure it, causing a more than 15% incremental spending increase on application and data security."
At the end of the webinar, Avivah Litan from Gartner emphasizes that AI security is key to consider in the AI Governance framework and cost. The main key issue to take-away is that "Investing in AI security risk and privacy tools increases security operational costs but also leads to more revenue growth."
While implementing TRiSM in the company is a real team effort, one essential part of its success is the employee education towards Change Management.
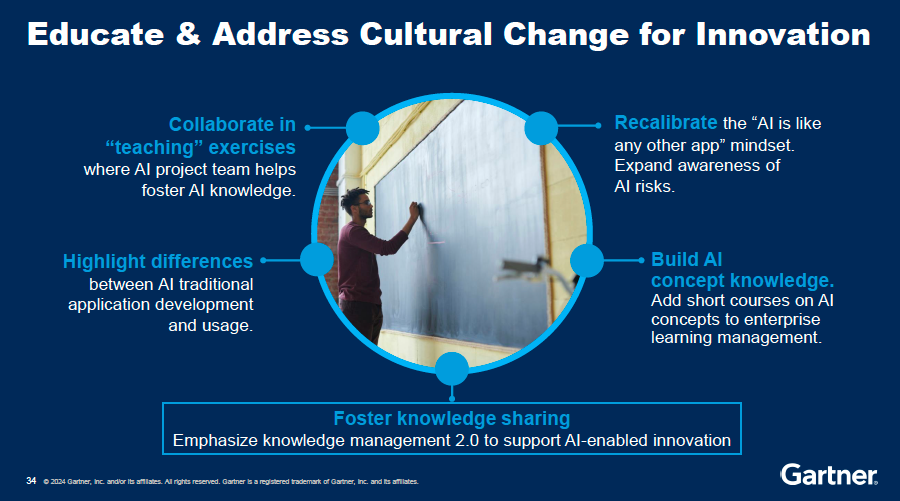
How to Build a Robust AI Security Strategy ?
To summarize what Gartner shared in this webinar, to effectively secure AI systems, organizations should consider :
- Establish centralized AI governance with clear budget authority
- Implement comprehensive data classification and access management
- Deploy AI-specific security tools for content anomaly detection
- Develop and enforce acceptable use policies
- Maintain ongoing monitoring and validation
35% More Revenue Growth
Investing in AI security might increase operational costs initially, but Gartner's research indicates that enterprises investing in TRiSM controls achieve 35% more revenue growth than those who don't. This demonstrates that proper AI security isn't just about risk mitigation.
Start NOW!
As AI continues to evolve, your company needs to adapt their security approaches.
Start by:
- Assessing your current AI security posture
- Identifying gaps in your security framework
- Implementing AI-specific security tools
- Training teams on new security protocols
- Establishing clear governance structures
Remember: AI security is a team sport requiring collaboration across IT, security, compliance, legal and business units. Success depends on building bridges between these traditionally siloed departments.
View the full webinar on Gartner´s website with Avivah Litan, Distingushed VP Analyst and Jeremy D´Hoinne, VP analyst.
Learn more about AI Security and AI Safety from our blog articles.
Dont´waste any more time, contact us for your company AI systems assessment.