Data Governance
The Foundation of Responsible AI Risk Management
In an era where AI systems are becoming increasingly prevalent, data governance has emerged as the cornerstone of responsible AI implementation.
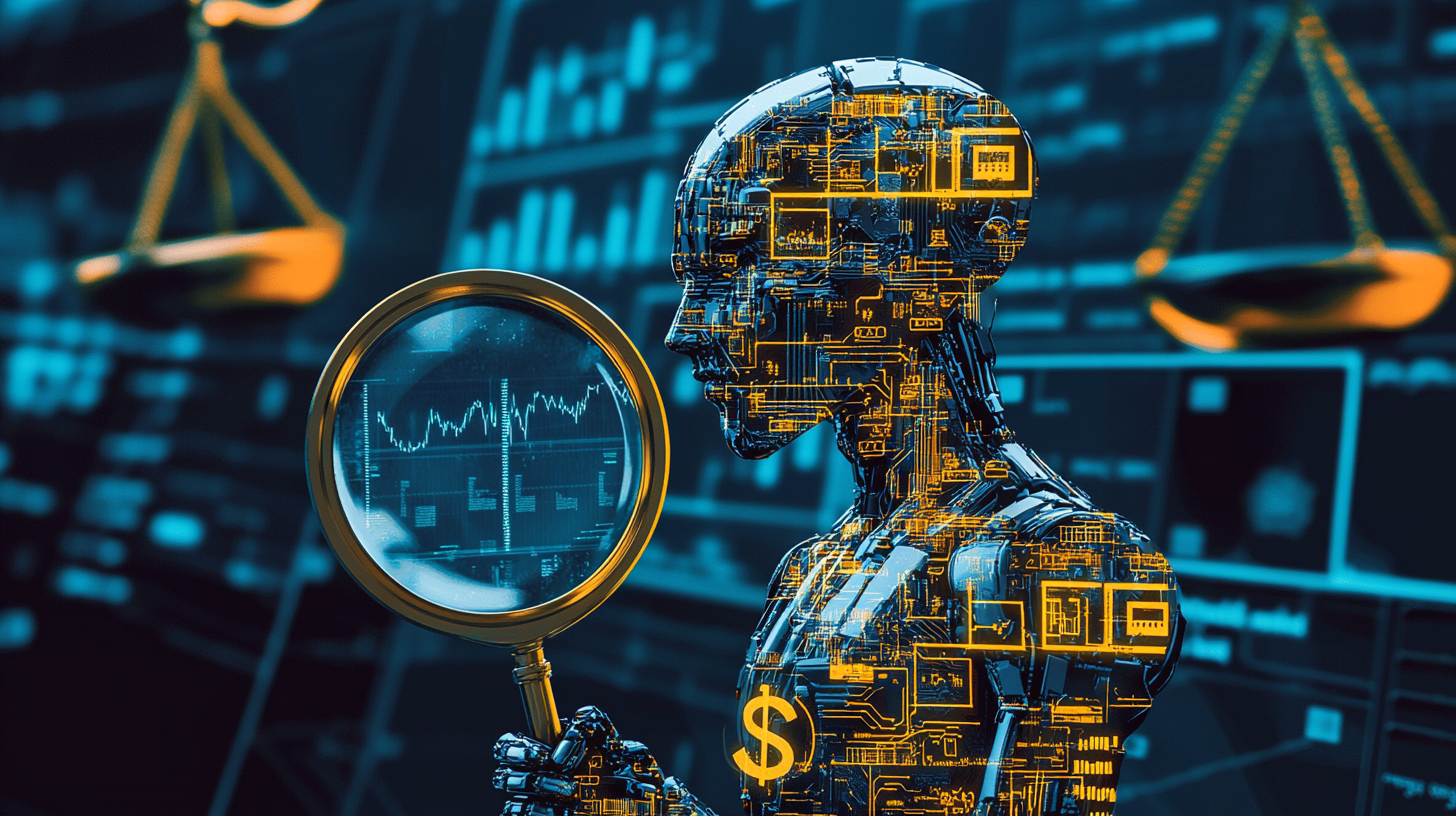
According to recent studies, 79% of organizations are approaching AI governance primarily through a data governance lens - and for good reason.
This comprehensive guide explores how organizations can leverage data governance frameworks to mitigate AI risks effectively while fostering innovation.
Picture this: You've just implemented a powerful AI tool like Microsoft's Copilot across your organization, expecting it to revolutionize your operations. Instead, you discover it's exposing sensitive HR data to unauthorized employees because of poor data management practices implemented during the rushed digital transformation of the COVID era. This scenario isn't fictional – it's a reality many organizations face today.
The Critical Role of Data in AI Governance
Data: The Lifeblood of Modern Organizations
In today's digital landscape, data isn't just an asset – it's the lifeblood of organizations. As Nina Bryant, Senior Managing Director at FTI Consulting, puts it, "Data sits at the heart of everything we do in organizations, but absolutely at the heart of everything we do with AI." This fundamental truth underpins why data governance has become crucial for mitigating AI risks.
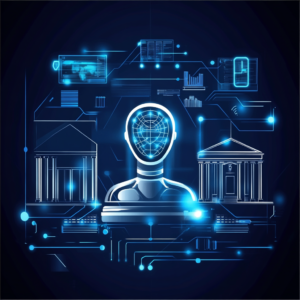
Why Data Governance Matters More Than Ever
Foundation for AI Success Training data quality directly impacts AI performance - this isn't just about having "clean" data. Organizations have discovered that AI systems trained on poorly managed data can amplify existing biases or make decisions based on outdated or incorrect information. For example, an AI recruitment tool might unfairly screen candidates if trained on historically biased hiring data. Data classification ensures appropriate usage - proper classification goes beyond simple categorization. It involves understanding data sensitivity levels, usage restrictions, and regulatory requirements. This becomes crucial when AI systems are processing multiple data types simultaneously. Data accuracy determines AI output reliability - in the age of AI, the old adage "garbage in, garbage out" has never been more relevant. When an AI system makes recommendations or decisions, the reliability of those outputs is directly tied to the accuracy of its training data.
Risk Mitigation Framework Prevents unauthorized data exposure - this is particularly critical in the context of AI systems that might inadvertently create connections between different data sets. For instance, a chatbot might accidentally reveal sensitive information by connecting seemingly unrelated data points. Reduces bias in AI systems - proper data governance includes regular audits and assessments of training data to identify and correct potential biases before they become embedded in AI decision-making processes. This includes both obvious and subtle forms of bias that might exist in historical data. Ensures compliance with regulations - as regulatory frameworks around AI continue to evolve, strong data governance provides the foundation for maintaining compliance. This includes documenting data lineage, managing consent, and ensuring appropriate data usage.
The Three Pillars of Data Governance for AI Risk Management
Antes de dominar la comunicación con IA, necesitamos entender con quién estamos hablando. Los Modelos de Lenguaje de Gran Escala (LLMs) son las mentes artificiales más avanzadas jamás creadas, capaces de procesar y generar texto de forma asombrosamente humana.
Strategic Data Management :
Implementation of robust data classification systems - this involves creating and maintaining a comprehensive taxonomy that reflects both business needs and regulatory requirements.
Organizations need to consider:
- Classification levels (public, internal, confidential, restricted)
- Data types (personal, financial, operational)
- Usage restrictions
- Retention requirements
- Regulatory implications
Clear data access controls - this goes beyond simple permission settings. Modern data access control should:
- Implement the principle of least privilege
- Include contextual access controls
- Monitor and log access patterns
- Regularly review and update permissions
- Integrate with identity management systems
Operational Excellence :
Regular data quality assessments - this should be a structured process that includes:
- Automated data quality checks
- Manual review processes
- Statistical analysis of data patterns
- Regular audits of data accuracy
- Feedback loops from AI system performance
Continuous monitoring of AI systems - this involves:
- Real-time performance monitoring
- Output validation
- Bias detection
- Anomaly detection
- User feedback analysis
Compliance and Ethics:
Organizations must ensure:
- Alignment with regulatory requirements (GDPR, AI Act, etc.)
- Ethical use of data in AI systems
- Transparent data handling practices
- Regular audits and assessments
Real-World Implementation Strategies
Starting Small: The TELUS Approach
TELUS, a leading telecommunications company, provides an excellent example of practical implementation. Their approach includes:
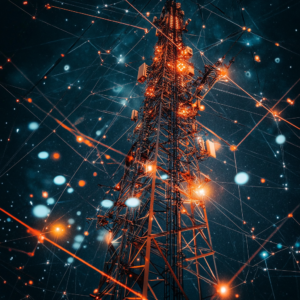
- Focused Initial Implementation
- Starting with internal use cases
- Building confidence through small wins
- Gradual expansion to customer-facing applications
- Cross-Functional Collaboration
- Formation of dedicated "squads"
- Integration of diverse perspectives
- Regular stakeholder engagement
- Continuous Learning and Adaptation
- Regular assessment of outcomes
- Iterative improvement of processes
- Knowledge sharing across teams
The Purple Team Approach to AI Risk Management
One of the most innovative approaches to emerge in recent years is the "Purple Team" methodology, combining:
- Red Team: Security experts testing for vulnerabilities
- Blue Team: Development team implementing safeguards
- Combined Approach: Collaborative risk identification and mitigation
Benefits of Purple Teaming
- Early risk identification
- Rapid response to issues
- Enhanced cross-team collaboration
- Improved AI literacy across the organization
- Better stakeholder engagement

Practical Steps for Implementation
Assessment Phase
Start with a comprehensive assessment of your current state:
- Data inventory analysis
- Existing governance framework review
- Risk assessment
- Stakeholder mapping

Planning Phase
Develop a structured implementation plan:
- Define clear objectives
- Set measurable KPIs
- Establish timelines
- Allocate resources
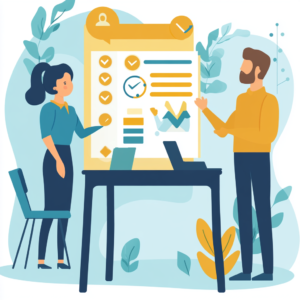
Implementation Phase
Execute your plan with:
- Pilot programs
- Regular checkpoints
- Stakeholder feedback
- Continuous adjustment

Addressing Common Challenges
Third-Party Vendor Management
When dealing with AI vendors:
- Request Essential Documentation
- Model cards
- Training data information
- Performance metrics
- Risk assessments
- Establish Clear Requirements
- Data handling protocols
- Security standards
- Performance expectations
- Compliance requirements

Building Internal Capabilities
Focus on developing:
- Technical expertise
- Process knowledge
- Risk awareness
- Collaborative skills

Future-Proofing Your Approach
Emerging Trends
Stay ahead by monitoring:
- Regulatory developments
- Technical standards evolution
- Industry best practices
- New risk management frameworks

Continuous Improvement
Maintain effectiveness through:
- Regular reviews
- Process updates
- Technology upgrades
- Team training

The Path Forward: Key Recommendations
Start with Fundamentals
- Build strong data governance foundations
- Establish clear policies and procedures
- Develop robust control frameworks

Focus on Integration
- Align with existing processes
- Build on current frameworks
- Leverage existing tools

Prioritize Collaboration
- Foster cross-functional teams
- Encourage knowledge sharing
- Build strong partnerships

Building Trust Through Governance
As organizations continue to adopt AI technologies, the importance of robust data governance cannot be overstated. It's not just about compliance or risk management – it's about building trust with stakeholders and ensuring sustainable innovation.
Ready to enhance your organization's data governance and AI risk management capabilities? we can help you develop and implement a comprehensive strategy tailored to your needs.
Schedule a Free Consultation to Assess Your Data Governance Needs.
Expert Panel Contributors
Nina Bryant Senior Managing Director in the Technology segment at FTI Consulting Head of UK Information Governance Team and Privacy & Security Practice Based in London, Nina brings extensive experience in data governance and AI risk management, helping organizations navigate the complex intersection of technology, privacy, and security.
Luisa Resmerita Senior Director of Information Governance, Privacy & Security at FTI Consulting A specialist in regulatory compliance and data protection, Luisa helps organizations develop and implement effective governance frameworks for emerging technologies.
Jessalyn Diamond Director of Data Ethics at TELUS A leader in Responsible AI and Data Innovation, Jessalyn brings a unique perspective as a trained librarian now working at the forefront of AI governance, focusing on practical implementation of ethical AI principles.
Moderated by Joe Jones The discussion was expertly moderated by Joe Jones, bringing together these industry leaders to share their insights and experiences in data governance and AI risk management.
This article was based on insights shared during an IAPP web conference called "Data governance approaches to mitigating AI risk" sponsored by FTI Consulting. The conference explored practical approaches to mitigating AI risks through effective data governance. Source : IAPP AI Governance in practice report 2024.