Are you among the 72% of companies relying on third-party AI tools for your organization ? As artificial intelligence becomes increasingly integral to business operations, the challenge of implementing these tools safely and effectively has never been more critical.
The Rise of Third-Party AI Implementation
Organizations are rapidly adopting 3rd party AI tools instead of developing in-house solutions, primarily due to resource constraints and competitive pressures. According to the IAPP and EY's Organizational AI Governance Report, while 72% of companies rely on third-party AI tools, only 46% develop AI solutions in-house.
"Companies are often adopting and using third-party tools to avoid a competitive disadvantage because they're arriving in applications that organizations are already using," explains Aveen Sufi, Senior Manager for Global Privacy and AI Governance Chair at Dexcom.
AI Governance Being Built on Top of Existing Processes
AI Governance should not be apart from all other departments. The key is to build upon and integrate with what already exists, rather than reinventing the wheel, in order to maintain agility and efficiency in the face of rapid AI development.
-
Leverage existing processes: it is recommended layering AI governance on top of existing organizational processes, rather than creating entirely new processes from scratch. This helps maintain agility and avoid overly cumbersome bureaucracy.
-
Identify entry points: look across the organization to identify all the potential entry points where AI vendors could be onboarded without proper oversight. Then work to "plug those holes" by integrating AI governance checks into existing procurement, legal, and other relevant processes.
-
Integrate with privacy/data governance: integrate AI governance questions and assessments into existing privacy impact assessments, data protection impact assessments and other data governance frameworks. This helps leverage existing structures.
-
Leverage existing stakeholders: rather than creating a new AI-specific committee, engage the cross-functional stakeholders (legal, compliance, IT, data, etc.) who are already involved in existing technology procurement and risk management processes.
-
Start with existing principles: while the landscape is evolving quickly, it is recommended to focus on established principles and frameworks (e.g. OECD AI principles) as a foundation rather than attempting to build something entirely new.
Starting with Internal Assessment
Before going into vendor selection, organizations are likely to conduct thorough internal assessments.
This first step helps:
Understanding your AI Landscape
- Identify existing AI implementations within your organization
- Map out also where AI is available but not yet deployed
- Understand potential integration points and data touch-points
- Define clear business requirements and use cases (what? why?) BEFORE buying the technology
"If you don't truly understand what your use case is and you don't know what you're solving for, there's no guarantee that bringing on a tool is going to help you suddenly solve all those problems," cautions Sufi.
Without properly assessing this AI landscape, your company could waste efforts and money.
Building Your AI Governance Framework for Third-Party Vendor
As mentioned before, rather than creating entirely new processes, organizations should leverage existing frameworks. Dera Nevin, Managing Director at FTI Consulting, emphasizes that AI governance should be viewed as a "thin veneer" over existing processes:
Key Components:
- Cross-functional oversight committees - multi-disciplinary exercise
- Integration with existing privacy and security assessments
- Clear vendor evaluation criteria
- Documented incident management procedures
- Ongoing monitoring protocols
Vendor Assessment and Due Diligence
When evaluating AI vendors, Tracy Bordignon, Senior Director at FTI Consulting, recommends focusing on:
Critical Assessment Areas Check-list
- Technology ownership (proprietary or third-party) and architecture
- Data quality and training/validating/testing methodologies
- Privacy compliance documentation regarding the region
- Model explainability
- Continuous monitoring and maintenance protocols by your own company
Proof of Concept Testing
Organizations should implement "proof-of-concept testing" before full deployment.
This approach helps:
-
- Validate business use cases
- Test data compatibility with limited set of users to check on model performance (risk mitigation)
- Assess user acceptance to assist for future employee training
- Identify potential risks
- Evaluate performance metrics
Ongoing Monitoring and Management
"AI is a living thing" emphasizes Bordignon.
Therefore, continuous monitoring is essential for:
- Detecting model drift
- Ensuring continued accuracy
- Maintaining regulatory compliance
- Identifying potential biases
- Validating performance against business objectives
Incident Management and Response
For this part, it is important to understand the root cause of any AI-related incidents or errors, whether it's an issue with the model, the data or the way the system is being used within the organization. This can help determine the appropriate mitigation strategies and assign responsibility accordingly.
-
Model failure refers to issues with the underlying AI model itself, such as the math or algorithms not working as expected. For example, issues with hallucinations in generative AI models where the output doesn't match the intended behavior.
-
Data failure refers to problems with the data used to train or power the AI system. The data may be biased, outdated, or otherwise unfit for the intended use case.
-
Use failure refers to misunderstandings or misuse of the AI system by the end user. The system may be working as intended, but the user prompts or uses it in a way that leads to unintended or problematic outcomes.
Your company must prepare for potential AI system risks.
Proactively build a framework in case on "what could go wrong ?" and identify :
-
- Clearly defining what constitutes an incident
- Establishing notification protocols
- Documenting response procedures
- Determining liability and responsibility
- Creating recovery plans
Looking Ahead
As AI regulation evolves and technology advances, your company should remain flexible and proactive in their governance approaches. "This is new enough that there's no gold standard for doing this anywhere," notes Nevin. "We are all working through this together."
While international standards and principles are pretty set, the laws may be evolving.
Best Practices for Success
- Leverage existing governance frameworks
- Maintain transparency across stakeholders
- Focus on clear use cases
- Account for total cost of ownership
- Invest in proper training and expertise
As a Conclusion
Implementing third-party AI tools requires a balanced approach between innovation and risk management. By following these guidelines and maintaining a strong governance framework, organizations can successfully navigate the complexities of AI implementation while ensuring compliance and effectiveness.
Also, organizations need to carefully consider and budget for the complete set of costs involved in implementing and operating an AI system, not just the technology itself :
- Organizations often underestimate the full lifecycle costs beyond just the technology costs:
- There are significant change management and workflow costs involved in upskilling and redeploying staff.
- Companies may need to hire more expensive subject matter experts (SMEs) to manage the AI system outputs, rather than just replacing lower-cost roles.
- These additional human capital and change management costs are often overlooked when budgeting for an AI implementation.
- Dera emphasized the importance of capturing these full lifecycle costs upfront, as they can have a big impact on the overall governance and success of the AI program.
An additional cost that is merely underestimated by companies is the security budget to secure their AI systems.
Remember: progress over perfection is the key to building a sustainable AI governance program that grows with your organization's needs and capabilities.
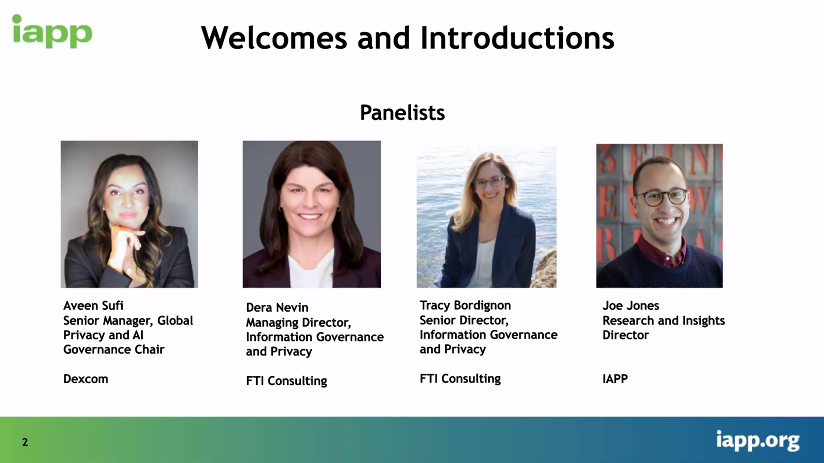
The panelist of this IAPP "Implementing third-party AI tools: Guardrails and vendor risk management" webinar were :
- Tracy Bordignon, AIGP, CIPM, Senior Director, Information Governance and Privacy, FTI Consulting
- Dera Nevin, CIPP/E, CIPP/US, CIPM, Managing Director, Information Governance and Privacy, FTI Consulting
- Aveen Sufi, CIPP/US, Senior Manager, Global Privacy and AI Governance Chair, Dexcom
- and Joe Jones, Research and Insights Director, IAPP as moderator.
View the full webinar at IAPP website.
Want to read more about AI Governance ? or contact us for an assessment for your company.